Understanding Data Annotation: A Comprehensive Guide
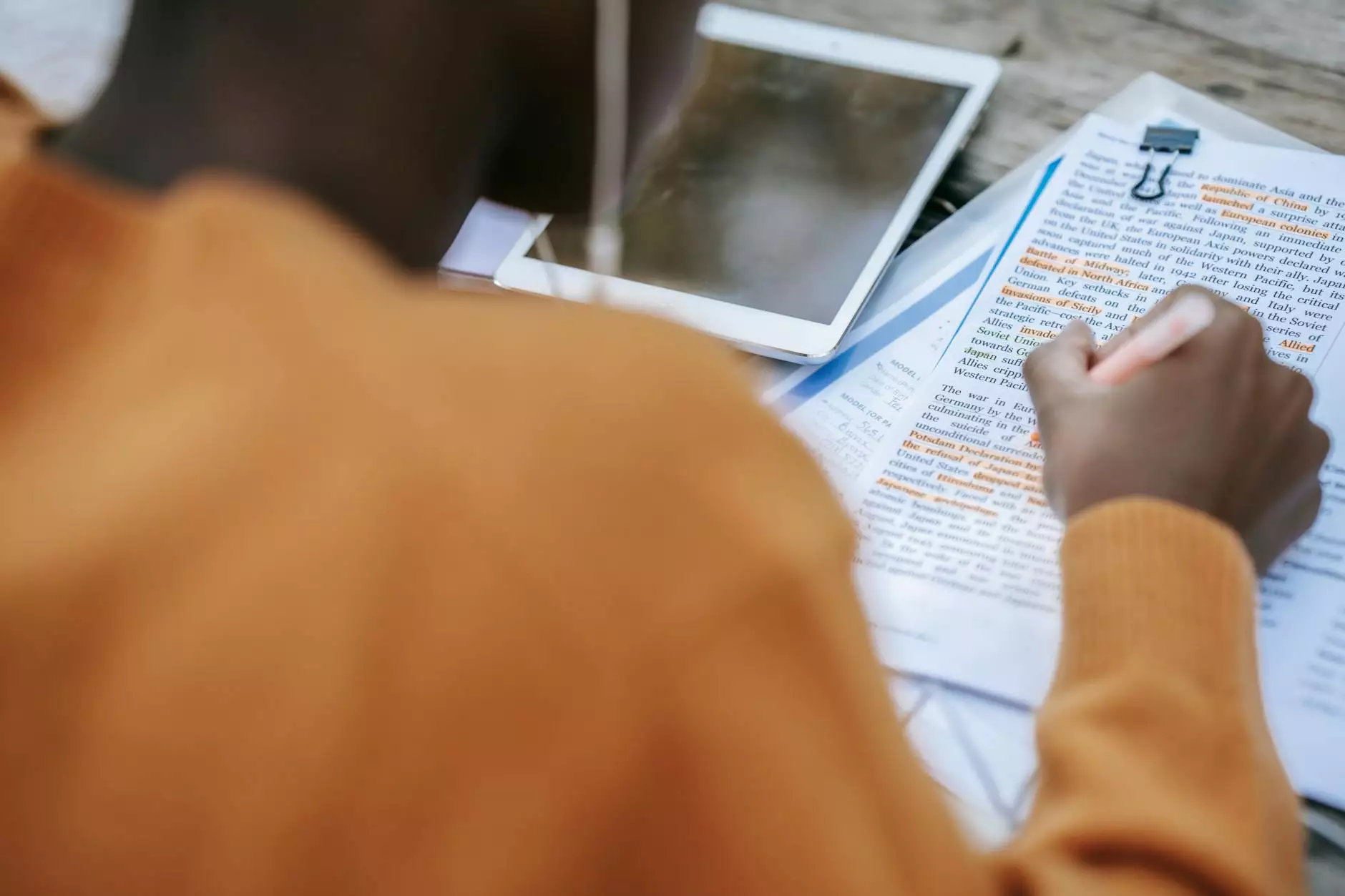
In today's technologically advanced world, data annotation has become a pivotal component of machine learning and artificial intelligence projects. It is the process that involves labeling data to train algorithms, ensuring that they can properly understand and process information. This article dives deep into the concept of data annotation, its significance, various types, and how businesses like keylabs.ai excel in providing top-notch data annotation tools and platforms to empower organizations.
What Is Data Annotation?
Data annotation refers to the act of labeling or tagging data to enhance its quality and usability, especially in machine learning models. By providing the necessary context, data annotation helps train algorithms to recognize patterns, categorize content, or take action based on input data. This process is critical in training AI systems to ensure accuracy, efficiency, and reliability.
The Importance of Data Annotation in Modern Business
The role of data annotation is crucial for various industries, including automotive, healthcare, finance, and more. Here are some significant impacts of data annotation on modern business:
- Quality Training Data: High-quality annotated data is essential for effective machine learning models. Data annotation ensures the model learns accurately from the information it processes.
- Improved AI Performance: Models trained on well-annotated data tend to perform better, providing more accurate predictions and classifications.
- Enhanced User Experience: Businesses leveraging AI can offer highly personalized services and products, leading to better customer satisfaction and loyalty.
- Faster Development Cycles: Efficient data annotation tools speed up the preparation of datasets, allowing organizations to launch their AI projects quicker.
Types of Data Annotation Techniques
There are various ways to annotate data, each suited to different types of machine learning tasks. Here, we outline some of the main types of data annotation techniques:
1. Image Annotation
Image annotation involves labeling objects or features in images, commonly used in computer vision tasks. Types of image annotation include:
- Bounding Boxes: Rectangles drawn around objects of interest.
- Image Segmentation: Pixel-wise labeling to identify the specific shape of objects.
- Landmark Annotation: Marking specific points of interest in images, such as facial features.
2. Text Annotation
Text annotation is critical for natural language processing (NLP) applications. This includes:
- Entity Recognition: Identifying names, dates, and locations within the text.
- Sentiment Analysis: Tagging text segments with positive, negative, or neutral sentiment.
- Part-of-Speech Tagging: Labeling words according to their function in the sentence.
3. Audio Annotation
Audio annotation is particularly valuable for speech recognition tasks. Techniques include:
- Transcribing: Converting spoken words into written text.
- Speaker Identification: Labeling audio clips with the speaker's identity.
- Emotion Detection: Annotating audio clips based on the emotion conveyed by the speaker.
Benefits of Using Data Annotation Tools and Platforms
Utilizing professional data annotation tools and platforms can significantly enhance the quality and efficiency of the annotation process. Here are the primary benefits:
1. Scalability
Platforms like keylabs.ai can handle large volumes of data, allowing businesses to scale their AI projects efficiently without compromising on quality.
2. Cost-Effectiveness
Automated and semi-automated annotation processes save both time and money, enabling businesses to focus on critical strategic elements instead of manual data handling.
3. Collaboration and Management
Data annotation platforms typically include tools to manage teams, track progress, and ensure consistency across annotations, streamlining workflows significantly.
KeyLabs.ai: A Leading Data Annotation Platform
KeyLabs.ai offers an unparalleled suite of data annotation tools designed specifically to meet the challenges faced by businesses today. Here are several standout features:
1. Advanced Annotation Features
The platform provides versatile annotation options, accommodating image, text, and audio data, ensuring all bases are covered for diverse machine learning tasks.
2. User-Friendly Interface
With an intuitive interface, users can easily navigate the platform, improving the overall user experience and reducing the learning curve for new team members.
3. Quality Assurance
KeyLabs.ai integrates advanced quality control mechanisms, utilizing machine learning algorithms to ensure that annotated data meets high standards.
4. Customizable Solutions
Recognizing that every business has unique needs, KeyLabs.ai offers customizable annotation solutions tailored to specific project requirements.
5. Dedicated Support
The platform provides responsive customer support, ensuring businesses can resolve any issues swiftly and maintain productivity.
Best Practices for Effective Data Annotation
- Define Clear Objectives: Establish what the end goal of the annotation is, guiding annotators effectively.
- Train Annotators Thoroughly: Providing comprehensive training for annotators ensures consistency and precision.
- Implement Quality Checks: Regularly review and validate annotations to maintain high standards of quality.
- Leverage Automation: Use automated tools where possible to improve efficiency and reduce time spent on repetitive tasks.
Conclusion
As machine learning continues to revolutionize industries, the importance of data annotation cannot be overstated. Utilizing advanced data annotation tools and platforms like keylabs.ai empowers businesses to streamline their AI initiatives, ensuring they remain competitive in a fast-evolving market. By investing in high-quality data annotation practices, companies can unlock the full potential of their data, driving informed decisions and fostering innovation. Embrace the future of AI and data management with effective data annotation strategies today.